Let's get real about AI
In recent years, artificial intelligence (AI) has been attracting more attention, money, and talent than ever in its short history. But much of the sudden hype is the result of myths and misconceptions being peddled by people outside of the field.
For many years, the field was growing incrementally, with existing approaches performing around 1-2 percent better each year on standard benchmarks. But there was a real breakthrough in 2012 when computer scientist Geoffrey Hinton and his colleagues at the University of Toronto showed that their “deep learning” algorithms could beat state-of-the-art computer vision algorithms by a margin of 10.8 percentage points on the ImageNet Challenge (a benchmark dataset).
At the same time, AI researchers were benefiting from ever-more-powerful tools, including cost-effective cloud computing, fast and cheap number-crunching hardware (“GPUs”), seamless data sharing through the internet, and advances in high-quality open-source software.
Owing to these factors, machine learning, and particularly deep learning, has taken over AI and created a groundswell of excitement. Investors have been lining up to fund promising AI companies, and governments have been pouring hundreds of millions of dollars into AI research institutes.
While further progress in the field is inevitable, it will not necessarily be linear. Nonetheless, those hyping these technologies have seized on a number of compelling myths, starting with the notion that AI can solve any problem.
Hardly a week goes by without there being sensational stories about AIs outperforming humans: “Intelligent Machines are Teaching Themselves Quantum Physics”; “Artificial Intelligence Better Than Humans at Spotting Lung Cancer.” Such headlines are often true only in a narrow sense.
For a general problem like “spotting lung cancer,” AI offers a solution only to a particular, simplified rendering of the problem, by reducing the task to a matter of image recognition or document classification.
What these stories neglect to mention is that the AI doesn’t actually understand images or language the way humans do. Rather, the algorithm finds hidden, complex combinations of features whose presence in a particular set of images or documents is characteristic of a targeted class (say, cancer or violent threats).
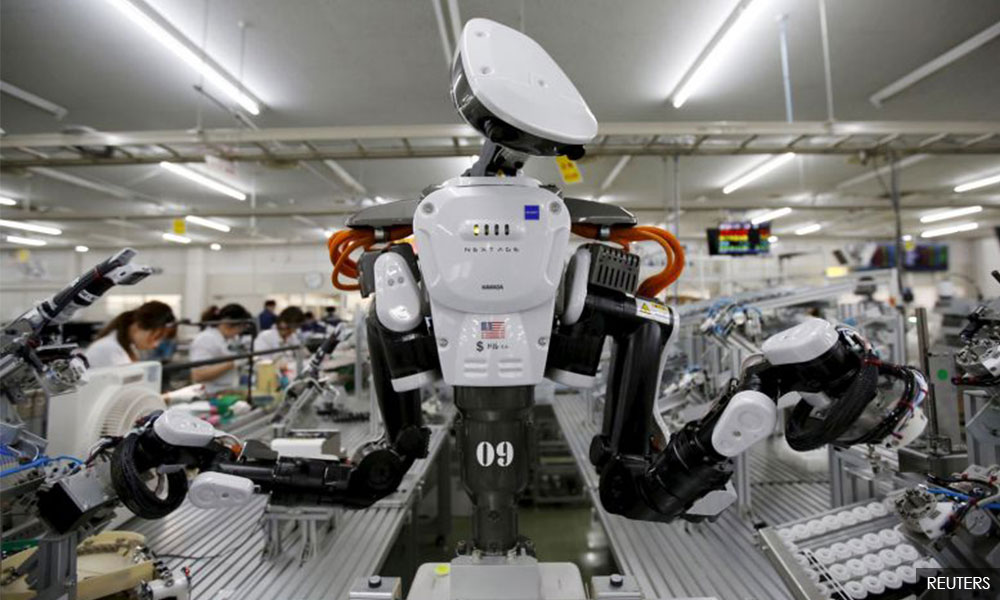
And such classifications cannot necessarily be trusted with decisions about humans – whether it concerns patient diagnosis or how long someone should be incarcerated.
It’s not hard to see why. Although AI systems outperform humans in tasks that are often associated with a “high level of intelligence” (playing chess, Go, or Jeopardy), they are nowhere close to excelling at tasks that humans can master with little to no training (such as understanding jokes). What we call “common sense” is actually a massive base of tacit knowledge – the cumulative effect of experiencing the world and learning about it since childhood.
Coding common-sense knowledge and feeding it into AI systems is an unresolved challenge. Although AI will continue to solve some difficult problems, it is a long way from performing many tasks that children undertake as a matter of course.
This points to a second, related myth: that AI will soon surpass human intelligence. In 2005, the best-selling futurist author Ray Kurzweil predicted that in 2045, machine intelligence will be infinitely more powerful than all human intelligence combined. But whereas Kurzweil was assuming that the exponential growth of AI would continue more or less unabated, it is more likely that barriers will arise.
Second-class citizens?
One such barrier is the sheer complexity of AI systems, which have come to rely on billions of parameters for training machine-learning algorithms from massive data sets. Because we already do not understand the interactions between all of these parts of the system, it is difficult to see how various components can be assembled and connected to perform a given task.
Another barrier is the scarcity of the annotated (“labelled”) data upon which machine-learning algorithms rely. Big Tech firms like Google, Amazon, Facebook, and Apple own much of the most promising data, and they have little incentive to make such valuable assets publicly available.
A third myth is that AI will soon render humans superfluous. In his best-selling 2015 book, Homo Deus: A Brief History of Tomorrow, Israeli historian Yuval Noah Harari argues that most humans may become second-class citizens of societies in which all higher-level intellectual decision-making is reserved for AI systems.
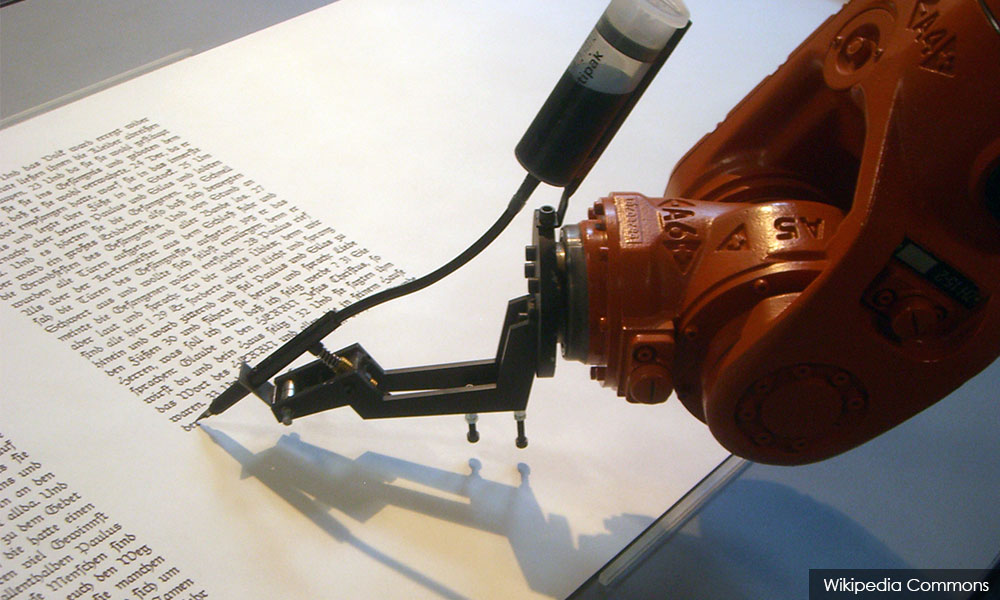
Indeed, some common jobs, such as truck driving, will most likely be eliminated by AI within the next ten years, as will many white-collar jobs that involve routine, repetitive tasks.
But these trends do not mean that there will be mass unemployment, with millions of households scraping by on a guaranteed basic income. The old jobs will be replaced by new jobs that we have yet to imagine. In 1980, no one could have known that millions of people would soon make a living from adding value to the internet.
To be sure, the jobs of the future will probably require much higher levels of math and science training. But AI itself may offer a partial solution, by enabling new, more engaging methods of training future generations in the necessary competencies.
Jobs that AI takes away will be replaced by new jobs for which AI trains people. There is no law of technology or history that destines humanity to a future of intellectual slavery.
There are of course more myths: AIs will overpower and harm humans, will never be capable of human-type creativity, and will never be able to build a causal, logical chain connecting effects with the patterns that cause them. I believe time and research eventually will debunk these myths as well.
This is an exciting time for AI. But that is all the more reason to remain realistic about the field’s future.
- Project Syndicate
STAN MATWIN, professor of computer science, Canada Research chair, and director of the Institute for Big Data Analytics at Dalhousie University in Halifax, Nova Scotia, is a professor at the Institute of Computer Science at the Polish Academy of Sciences. - Mkini
The views expressed here are those of the author/contributor and do not necessarily represent the views of MMKtT.
✍ Credit given to the original owner of this post : ☕ Malaysians Must Know the TRUTH
🌐 Hit This Link To Find Out More On Their Articles...🏄🏻♀️ Enjoy Surfing!
Post a Comment